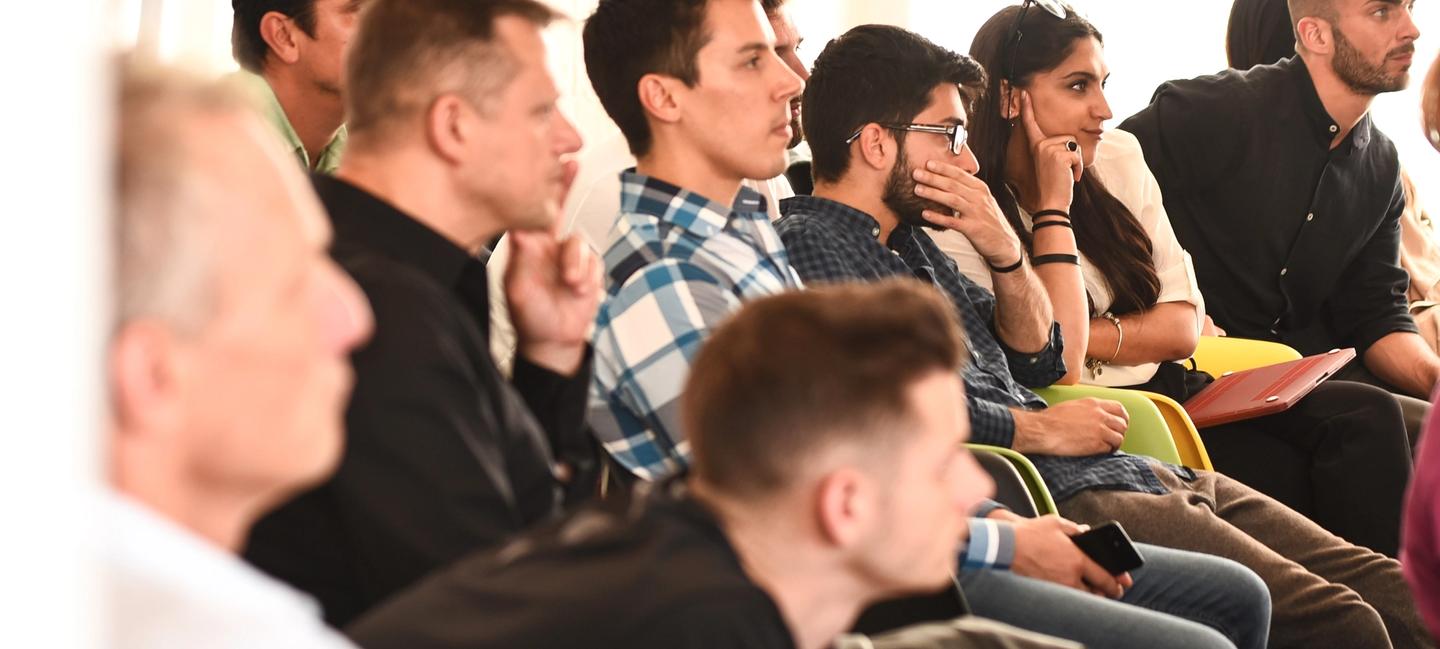
Seminar: Peter Frazier - Cornell University & Uber
Knowledge-Gradient Methods for Grey-Box Bayesian Optimization
Abstract
The knowledge gradient (KG) is a class of Bayesian optimization acquisition functions that unlocks significant value and flexibility. While expected improvement is effective for standard Bayesian optimization, its guidance becomes questionable when we can measure quantities that don't directly create improvement but are obviously quite informative. Informative quantities undervalued by expected improvement include gradients, biased low-fidelity fast approximations of the objective function, and context variables that are unknown until measured. We provide an introduction to the KG acquisition function focusing on how it can be used in these more exotic Bayesian optimization settings. We then show how KG can be implemented easily to leverage GPU resources within BoTorch using the one-shot KG approach.
Notes
- BOTORCH: Bayesian Optimization in PyTorch https://arxiv.org/pdf/1910.06403.pdf
- Multi-Information Source Optimization, NeurIPS 2017 https://arxiv.org/abs/1603.00389
- Practical Multi-fidelity Bayesian Optimization for Hyperparameter Tuning, UAI 2019 https://arxiv.org/abs/1903.04703
- Peter Frazier is an Associate Professor at Cornell University in the School of Operations Research and Information Engineering, and Staff Data Scientist at Uber.