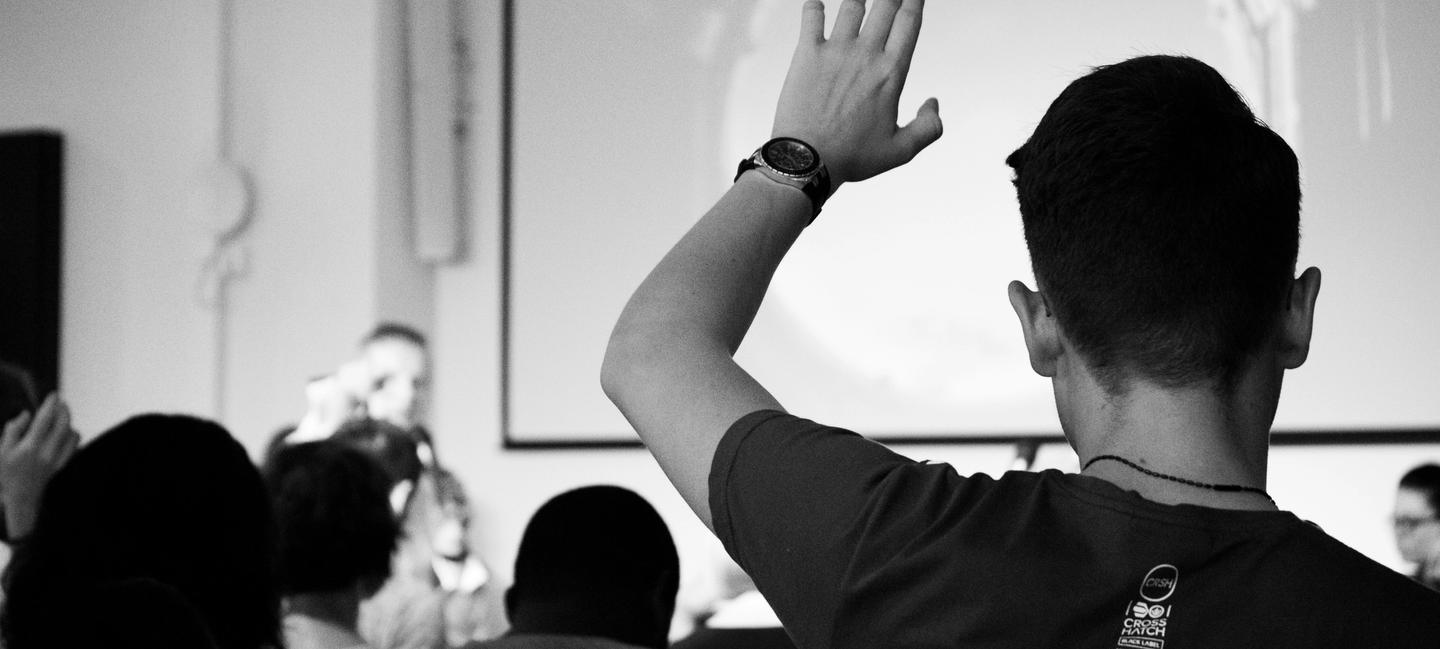
Seminar: Christopher Nemeth - University of Lancaster
Coin Sampling: Gradient-Based Bayesian Inference without Learning Rates
Abstract
In recent years, particle-based variational inference (ParVI) methods such as Stein variational gradient descent (SVGD) have grown in popularity as scalable methods for Bayesian inference. Unfortunately, the properties of such methods invariably depend on hyperparameters such as the learning rate, which must be carefully tuned by the practitioner in order to ensure convergence to the target measure at a suitable rate. In this talk, I will show how ideas from the learning-rate free optimisation literature can be used to create a suite of new particle-based methods for scalable Bayesian inference. By leveraging the view of sampling as optimisation on the space of probability measures, we can establish convergence of our approach in Kullback-Leibler (KL) divergence, and obtain non-asymptotic convergence rates when the target measure is (strongly) log-concave. I will illustrate the performance of this new coin sampling approach on a range of numerical examples, including several high-dimensional models and datasets, demonstrating comparable performance to other ParVI algorithms.
Notes
- References:
- Personal website can be found here .