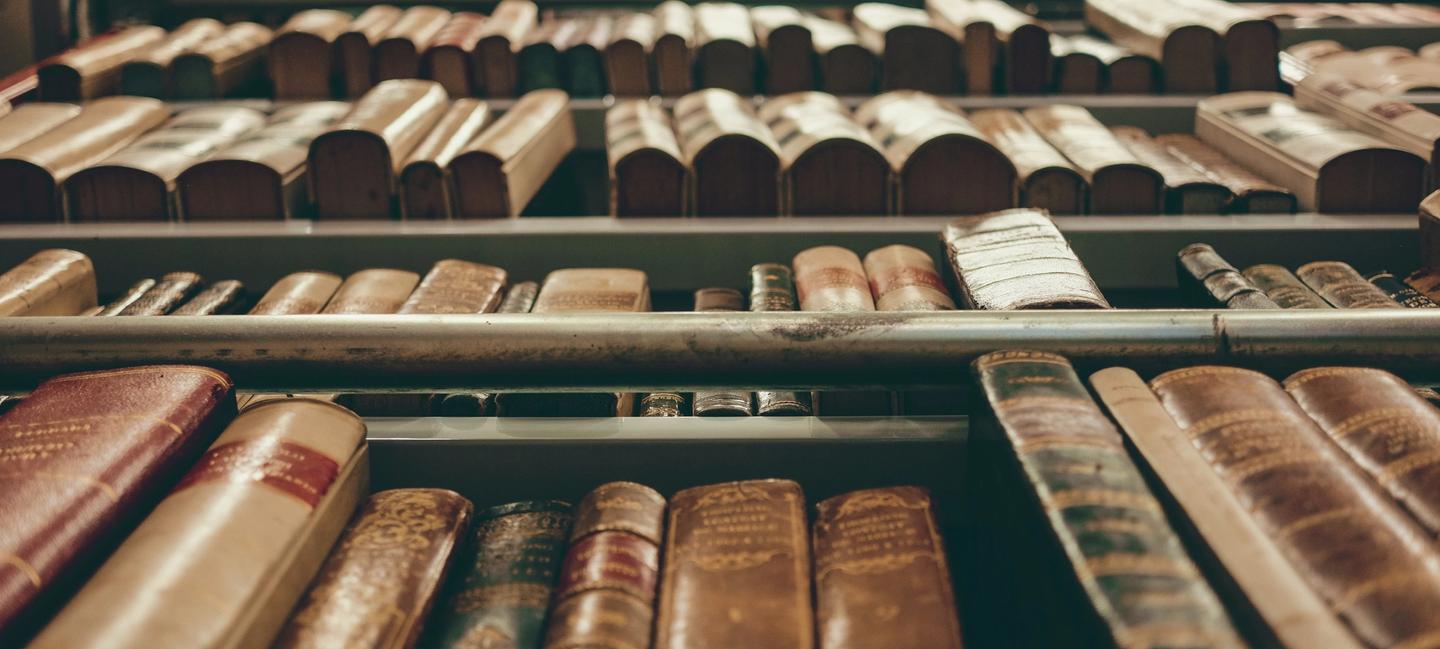
Reinforcement Learning in POMDPs
Many real-world reinforcement learning problems have both a hierarchical nature, and exhibit a degree of partial observability. While hierarchy and partial observability are usually tackled separately (for instance by combining recurrent neural networks and options), this paper proposes an integrated approach to deal with both issues at the same time. To achieve this, we extend the options framework for hierarchical learning to make the option initiation sets conditional on the previously-executed option. We show that options with such Option-Observation Initiation Sets (OOIs) are at least as expressive as Finite State Controllers. We also empirically demonstrate that OOIs are much more sample-efficient than using a recurrent neural network over options, and illustrate the flexibility of OOIs regarding the amount of domain knowledge available at design time.