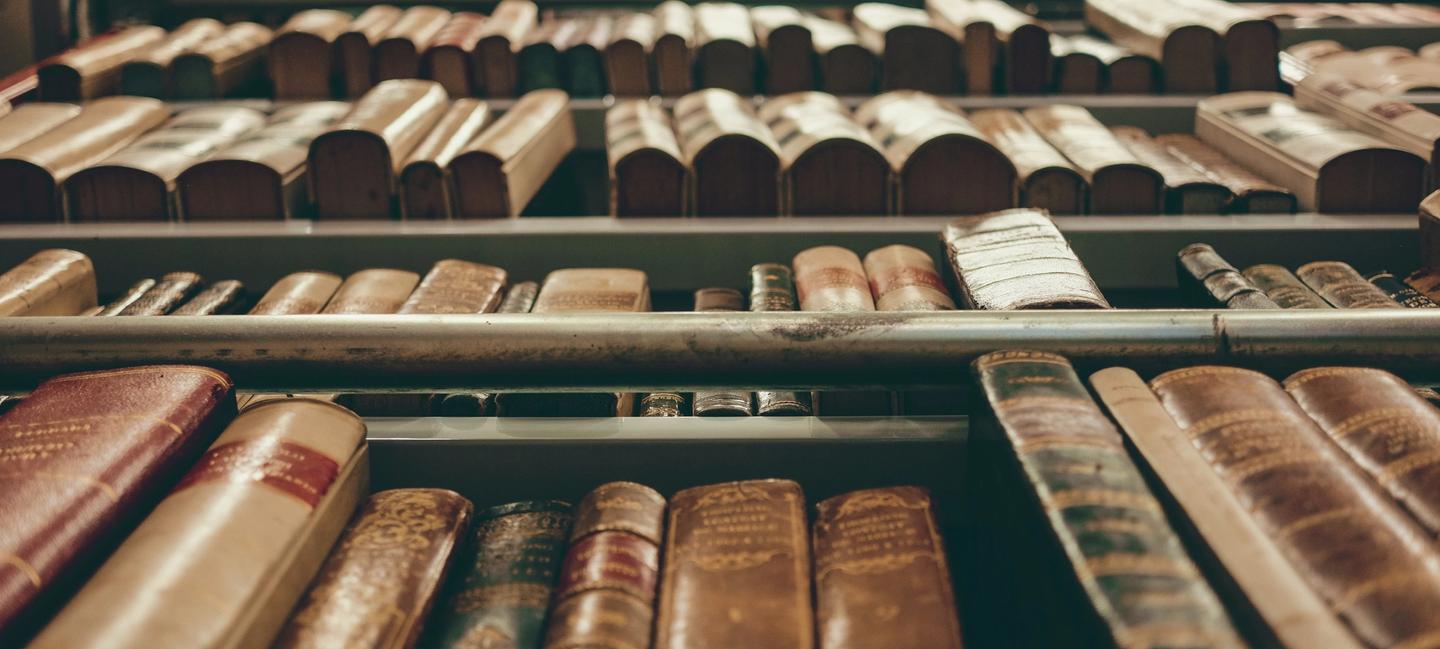
Modeling and Optimization with Gaussian Processes in Reduced Eigenbases
Parametric shape optimization aims at minimizing an objective function f(x) where x are CAD parameters. This task is difficult when f(·) is the output of an expensive-to-evaluate numerical simulator and the number of CAD parameters is large.Most often, the set of all considered CAD shapes resides in a manifold of lower effective dimension in which it is preferable to build the surrogate model and perform the optimization.In this work, we uncover the manifold through a high-dimensional shape mapping and build anew coordinate system made of eigenshapes. The surrogate model is learned in the space of eigenshapes: a regularized likelihood maximization provides the most relevant dimensions for the output. The final surrogate model is detailed (anisotropic) with respect to the most sensitive eigenshapes and rough (isotropic) in the remaining dimensions. Last, the optimization is carried out with a focus on the critical dimensions, the remaining ones being coarsely optimized through a random embedding and the manifold being accounted for through a replication strategy. At low budgets, the methodology leads to a more accurate model and a faster optimization than the classical approach of directly working with the CAD parameters.