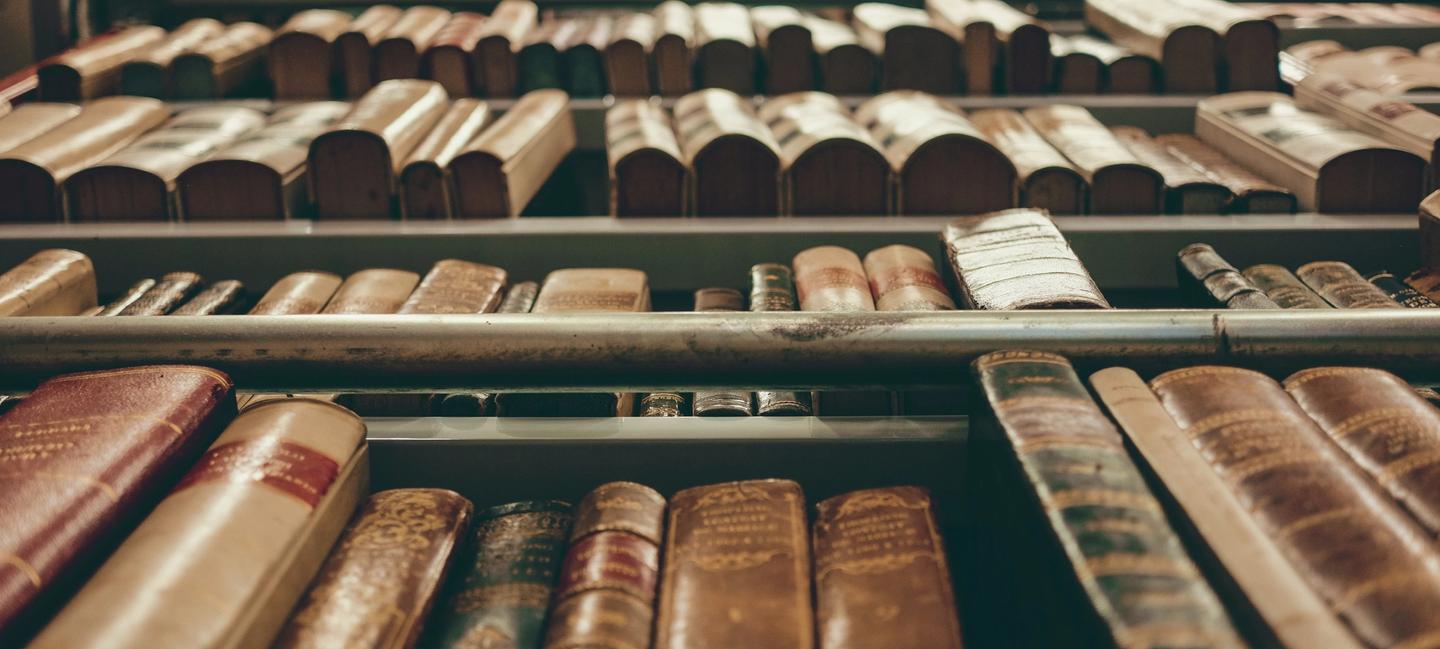
Inducing Point Allocation for Sparse Gaussian Processes
Full title: Inducing Point Allocation for Sparse Gaussian Processes in High-Throughput Bayesian Optimization
Sparse Gaussian Processes are a key component of high-throughput Bayesian Optimization (BO) loops; however, we show that existing methods for allocating their inducing points severely hamper optimization performance. By exploiting the quality-diversity decomposition of Determinantal Point Processes, we propose the first inducing point allocation strategy designed specifically for use in BO. Unlike existing methods which seek only to reduce global uncertainty in the objective function, our approach provides the local high-fidelity modeling of promising regions required for precise optimization. More generally, we demonstrate that our proposed framework provides a flexible way to allocate modeling capacity in sparse models and so is suitable for a broad range of downstream sequential decision-making tasks.